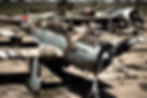
Introduction
It wasn't until early 2023 that I realized just how large and detrimental an impact cognitive biases can have on our/my analytical work. It took a whopping 12 years into my professional career for it to really hit home. It was a moment in my career when I realized just how much I still had to learn in order to help my team and future team's take their work to the next level and be better as a result for having me as their leader, versus anyone else.
The catalyst for this mid-career epiphany was Bhagyesh Phanse, our Vice President of Retail Consumer Analytics at CVS Health. He pulled his analysts and team leaders together for a focused session on biases - with the objective of making us aware of these biases, so that we in turn could confront and eliminate them in future work.
There were plenty of lessons and ah-ha moments that day, but today - we're focusing on one specific bias that we're all guilty of, and if we're not careful it has the ability to destroy our analysis, credibility, and reputation as analytical thinkers and leaders.
I've been in analytics for well over 10 years, leading analytics and data science teams for a good portion of that time. Here's the article that I wish someone had shared with me ten years ago...
Setting the Scene
Imagine this. It's the early 1940's, the world is at war for a second time and you're part of the Statistical Research Group, a team of mathematicians assembled to support the United States military. Your mission, is to help the military improve the survivability of the nation's military bomber planes. If you're successful, you'll be saving the lives of thousands of US and allied pilots. If you're unsuccessful, more fatalities will occur that you personally could have helped avoid. No pressure or anything!
You know that in order to improve the survivability of bombers, you'll need to get your hands on a high quality dataset in which to ground your research and conduct your study.
Their Bulletproof Test Plan
As a member of this exceptional research group, you have at your disposal some considerable resources, and luckily for you the military has been keeping meticulous records of the damage dealt to planes when they return from a mission. As the plane lands at the air base, it's ushered into the maintenance hangar and repair crews take pictures of the plane. The pictures are then added to the flight report and stored in the official military records.
You have a fantastic foundation here - all you need to do is take the pictures and translate the images into data. The team rolls up their sleevs and begins plotting all the bullet holes from each returning bomber on a diagram. A heat map of damage is taking shape.
You have hundreds of thousands of bullet hole location data points from tens of thousands of aircraft. You have a high level of confidence in the overall quality and scope of your dataset. The source of the data is the meticulous records kept by the US military, so you have no concerns with the cleanliness or credibility of the data. With all the damage in pictures and reports translated into data points, you can now identify the areas of US bombers that need to be reinforced to be more resilient in aerial combat.
Applying your Insights
Armed with some clear hot spots from your damage data, the team recommends immediate reinforcement of several areas of considerable concern. The team recommends to the military that they immediately reinforce and retrofit every bomber in the fleet in as follows:
Reinforce the perimeter of each wing
Add additional reinforcement to the underbelly of all aircraft
The military takes the recommendations seriously and jumps into action - retrofitting and reinforcing all aircraft based on your insights and recommendations. This couldn't be going any better! You assembled your data and cleansed it appropriately. You evaluated it exceptionally well. And you even effectively translated your learnings into actionable insights and recommendations - of which, you received complete and unanimous buy-in.
In the world of analytics - this is about as perfect as it gets. A rare perfect game.
The Problem
Despite the stellar insights, exceptional recommendations, and the quick and willing partnership of the military's engineers and leadership - after several months, the survivability of the reinforced bombers sent on missions is not improving. Not even by a single basis point.
The good news is that the survivability rate has not declined, but it hasn't improved either.
Eureka!
There's a gentleman in your research group with you. An exceptional mathematician named Abraham Wald. He has a radical idea. In a moment of clarity, bravery, and serendipitous inspiration - he says to the team "what if instead of reinforcing the areas that show the most damage, we reinforce the areas with the least damage?"
This is the part where puzzled looks emerge from everyone around the table. What's Abraham thinking here? Maybe he's been working on this project for too long and is starting to lose the ability to think critically and credibly.
Seeing everyone's puzzled look, Abraham explains his seemingly crazy idea.
"Our dataset is not flawed, it's simply incomplete. It does an exceptional job of showing us the damage that surviving planes incur, but it lacks any record of the places that destroyed or downed planes have sustained damage in combat. We're acting on excellent, but incomplete data."
Immediately the puzzled looks start to dissipate. They give way to excited grins and nods of agreement. The team is bought in.
Without access to the data from destroyed planes, the team makes an inspired (albeit partially uninformed) decision. They recommend that the hulls of bombers are reinforced in the areas where their data shows no significant or consistent damage. Instead of reinforcing the perimeters of the wings, the team recommended reinforcing areas around the fuselage, engines, and cockpit.
Real-World Application
Survivor bias occurs when we draw conclusions from an incomplete set of data. Specifically a set of data that has "survived" or passed through a specific selection or cleansing process... something that in the era of big data, almost every single analysis will have to go through.
The point where we're most prone to survivor bias is when we start applying constraints or parameters to filter or assemble an initial dataset.
Translating this into a specific scenario
You're asked to assess which of two specific products is more likely to create long-term retention in your brand. Your objective is to understand whether or not a consumer purchasing a specific item from you has any correlation to your ability to retain them in your broader business (i.e., if a consumer purchases X, they become Y% more likely to continue shopping with us).
Many times, organizations have standard parameters they use for audience selection (e.g., executives only care about consumers that have shopped more than once, customers must be currently active, etc.) - it's critical that you examine these for survivor bias.
If you perform your analysis and conclude that customers that shopped Product A were 23% more likely to keep shopping your brand than customers that shopped Product B - you may recommend that Product A be given more promotional resources than Product B. Your conclusion turns out to be accurate, albeit flawed, as you complied with your organization's standard approach to only include customers that have been active in the last two years.
By only looking at customers who have been active in the last two years, you've eliminated the "non-survivors" from your dataset - which could in-turn have been disproportionately engaged with Product A. Once you factor in these churned customers to Product A's retention rate, you realize that Product B is actually 8% more effective at securing year-over-year retention vs. Product A.
Your insight and recommendation has just changed by 180 degrees.
Six questions to ask to prevent survivor bias
Guarding against survivor bias doesn't require an advanced degree or any atypical thinking. You can start by asking yourself or your team these questions before moving on to the evaluation phase of your analysis.
Are we considering the whole picture? Does the data contain both successful and unscuccessful outcomes - or does it focus on the subset of positive results?
What data are we missing? Explore whether there is information about failures or non-survivors that might have been omitted. No finger pointing!
Is the sample representative? Determine whether the data sample accurately represents the entire population that you're analyzing, or if it disproportionately (or wholly) represents certain subsets.
Have we accounted for selection bias? Consider whether the data collection process introduces any bias on the selection criteria.
Are we learning from failures/non-survivors? Ensure that the analysis includes insights derived from failed or unsuccessful attempts at a specific outcome that you're looking to test for.
What assumptions are we making? Review the assumptions made regarding the analysis and determine whether or not they may inadvertantly favor specific outcomes.
Pro tip: Always list out any assumptions made and selection criteria applied when presenting your findings and/or your proposed analytical agenda/approach. If you're a leader, invest extra time upfront in the development of an analytical agenda to ensure that survivor bias (and other biases) have been eliminated from the action plan before your team invests their own valuable time on pursuing insights.
Call to Action
Share this article with an analyst or analytical leader/thought partner who takes great pride in their work, but is frequently asked to embed specific constraints or parameters into their work from their leadership team, stakeholders, or partners.